19/09/2024 UWB Respiratory rate monitoring
In a master's thesis by Anton Lambrecht, under supervision of Stijn Luchie and Eli De Poorter, machine learning (ML) techniques and algorithms were developed to determine the breathing rate of persons based on Ultra-WideBand (UWB) data.
To validate the algorithms and ML techniques data was captured in the realistic HomeLab environment.
Participants engaged in a series of measurements in different rooms. The scenarios included sitting on a sofa, laying on bed, standing up, etc. In these scenarios they were asked to breathe at a certain rate. This breathing rate was simultaneously captured by a ground-truth chest-strap device and a UWB transmitter-receiver pair.
The results indicate that it is possible to determine the breathing rate of a person, both with ML techniques and with conventional algorithms. Nonetheless, there is still much room for improvement. As expected, laying down in bed gives the most reliable and accurate results.
This research contributes to the broader understanding of how IoT devices such as UWB, in the past mainly used for localization, can be re-used for other applications such as vital-sign monitoring, object detection, etc. This results in an overall lower power consumption and lower operational cost.
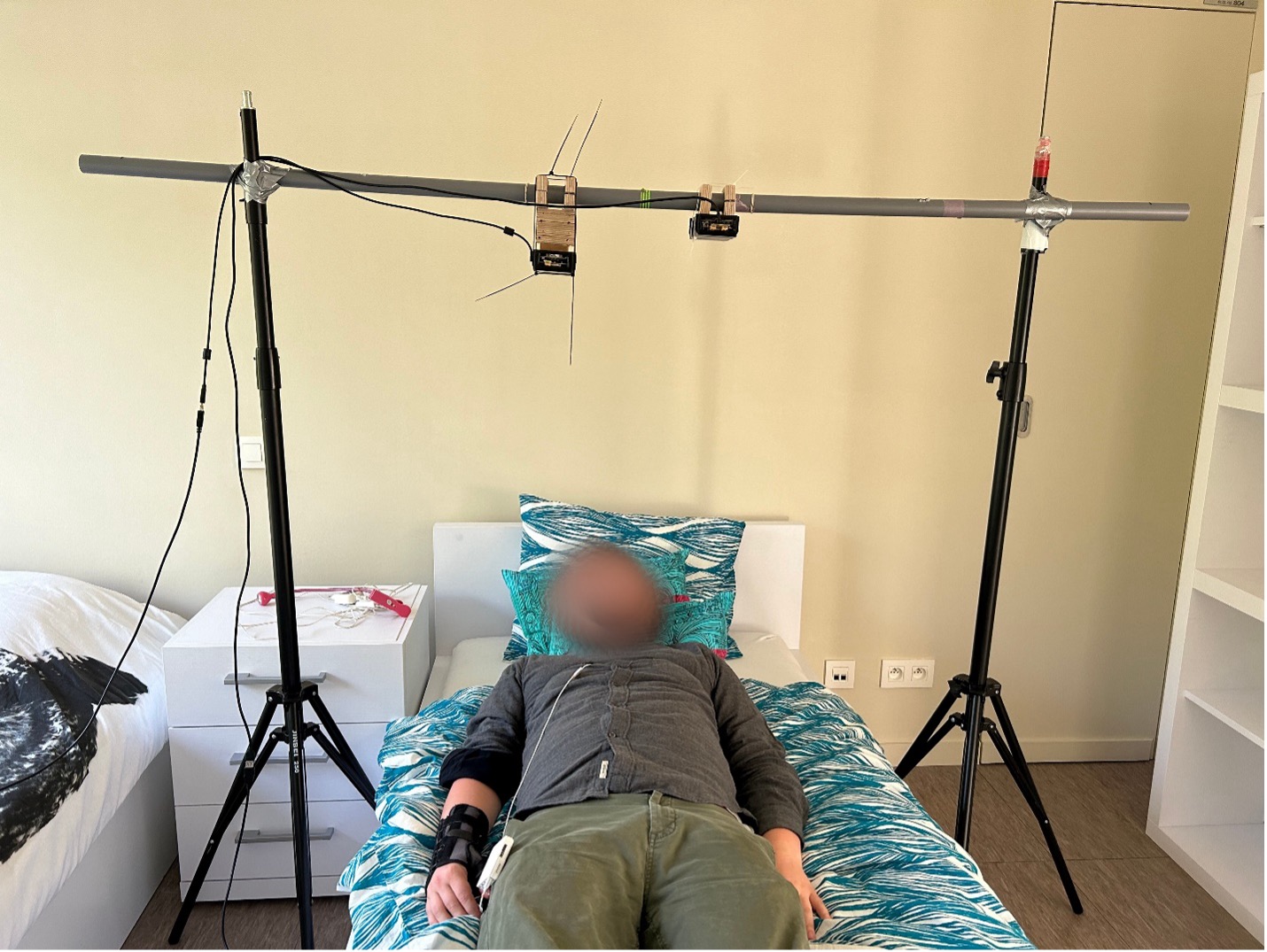